Research Replication: Unsupervised Learning of Digit Recognition Using STDP (Part 2)#
In our last tutorial, we designed an SNN to classify digits. We used the MNIST dataset for training. However, we used a scaled down model for illustration. In this notebook, we’re going to scale up the model a bit (though not to the full scale in the paper). We are going to use 400 excitatory and inhibitory neurons, 28x28 digits, and pre-trained weights (from here).
First, let’s take a look at what our pre-trained STDP weights look like. Below, in a grid, we can see what the input weights for all 400 neurons look like.
Show code cell content
from myst_nb import glue
import numpy as np
np.random.seed(0)
NUM_NEURONS = 400
NUM_NEURON_COLS = 20
NUM_NEURON_ROWS = 20
DIGIT_WIDTH =28
DIGIT_HEIGHT=28
DIGIT_SIZE=DIGIT_WIDTH*DIGIT_HEIGHT
glue("NUM_NEURONS", NUM_NEURONS, display=False)
glue("NUM_NEURON_COLS", NUM_NEURON_COLS, display=False)
glue("NUM_NEURON_ROWS", NUM_NEURON_ROWS, display=False)
glue("DIGIT_SIZE", DIGIT_SIZE, display=False)
glue("DIGIT_WIDTH", DIGIT_WIDTH, display=False)
glue("DIGIT_HEIGHT", DIGIT_HEIGHT, display=False)
def poisson_fire(values, min_value=0, max_value=255, min_rate=0, max_rate=10, dt=0.001):
relativeValues = (values - min_value) / (max_value - min_value)
relativeRates = min_rate + relativeValues * (max_rate - min_rate)
probsOfFire = relativeRates * dt
firings = np.random.rand(*values.shape) < probsOfFire
return firings / dt
class SynapseCollection:
def __init__(self, n=1, tau_s=0.05, t_step=0.001):
self.n = n
self.a = np.exp(-t_step / tau_s) # Decay factor for synaptic current
self.b = 1 - self.a # Scale factor for input current
self.voltage = np.zeros(n) # Initial voltage of neurons
def step(self, inputs):
self.voltage = self.a * self.voltage + self.b * inputs
return self.voltage
class STDPWeights:
def __init__(self, numPre, numPost, tau_plus = 0.03, tau_minus = 0.03, a_plus = 0.1, a_minus = 0.11, g_min=0, g_max=1):
self.numPre = numPre
self.numPost = numPost
self.tau_plus = tau_plus
self.tau_minus = tau_minus
self.a_plus = a_plus
self.a_minus = a_minus
self.x = np.zeros(numPre)
self.y = np.zeros(numPost)
self.g_min = g_min
self.g_max = g_max
self.w = np.random.uniform(g_min, g_max, (numPre, numPost)) / numPost # Initialize weights
def step(self, t_step):
self.x = self.x * np.exp(-t_step/self.tau_plus)
self.y = self.y * np.exp(-t_step/self.tau_minus)
def updateWeights(self, preOutputs, postOutputs):
self.x += (preOutputs > 0) * self.a_plus
self.y -= (postOutputs > 0) * self.a_minus
alpha_g = self.g_max - self.g_min # Scaling factor for weight updates
preSpikeIndices = np.where(preOutputs > 0)[0] # Indices of pre-synaptic spiking neurons
postSpikeIndices = np.where(postOutputs > 0)[0]
for ps_idx in preSpikeIndices:
self.w[ps_idx] += alpha_g * self.y
self.w[ps_idx] = np.clip(self.w[ps_idx], self.g_min, self.g_max)
for ps_idx in postSpikeIndices:
self.w[:, ps_idx] += alpha_g * self.x
self.w[:, ps_idx] = np.clip(self.w[:, ps_idx], self.g_min, self.g_max)
class LIF:
def __init__(self, n=1, dim=1, tau_rc=0.02, tau_ref=0.002, v_th=1,
max_rates=[200, 400], intercept_range=[-1, 1], t_step=0.001, v_init = 0):
self.n = n
# Set neuron parameters
self.dim = dim # Dimensionality of the input
self.tau_rc = tau_rc # Membrane time constant
self.tau_ref = tau_ref # Refractory period
self.v_th = np.ones(n) * v_th # Threshold voltage for spiking
self.t_step = t_step # Time step for simulation
# Initialize state variables
# self.voltage = np.ones(n) * v_init # Initial voltage of neurons
self.voltage = np.random.uniform(0, 1, n) # Initial voltage of neurons
self.refractory_time = np.zeros(n) # Time remaining in refractory period
self.output = np.zeros(n) # Output spikes
# Generate random max rates and intercepts within the given range
max_rates_tensor = np.random.uniform(max_rates[0], max_rates[1], n)
intercepts_tensor = np.random.uniform(intercept_range[0], intercept_range[1], n)
# Calculate gain and bias for each neuron
# self.gain = self.v_th * (1 - 1 / (1 - np.exp((self.tau_ref - 1/max_rates_tensor) / self.tau_rc))) / (intercepts_tensor - 1)
# self.bias = np.expand_dims(self.v_th - self.gain * intercepts_tensor, axis=1)
self.gain = np.ones(n)
self.bias = np.zeros(n)
# Initialize random encoders
# self.encoders = np.random.randn(n, self.dim)
# self.encoders /= np.linalg.norm(self.encoders, axis=1)[:, np.newaxis]
self.encoders = np.ones((n, self.dim))
def reset(self):
# Reset the state variables to initial conditions
self.voltage = np.zeros(self.n)
self.refractory_time = np.zeros(self.n)
self.output = np.zeros(self.n)
def step(self, inputs):
dt = self.t_step # Time step
# Update refractory time
self.refractory_time -= dt
delta_t = np.clip(dt - self.refractory_time, 0, dt) # ensure between 0 and dt
# Calculate input current
I = np.sum(self.bias + inputs * self.encoders * self.gain[:, np.newaxis], axis=0) / self.n
# Update membrane potential
self.voltage = I + (self.voltage - I) * np.exp(-delta_t / self.tau_rc)
# Determine which neurons spike
spike_mask = self.voltage > self.v_th
self.output[:] = spike_mask / dt # Record spikes in output
# Calculate the time of the spike
t_spike = self.tau_rc * np.log((self.voltage[spike_mask] - I[spike_mask]) / (self.v_th[spike_mask] - I[spike_mask])) + dt
# Reset voltage of spiking neurons
self.voltage[spike_mask] = 0
# Set refractory time for spiking neurons
self.refractory_time[spike_mask] = self.tau_ref + t_spike
return self.output # Return the output spikes
class ALIF:
def __init__(self, n=1, dim=1, tau_rc=0.02, tau_ref=0.002, v_th=1,
max_rates=[200, 400], intercept_range=[-1, 1], t_step=0.001, v_init = 0,
tau_inh=0.05, inc_inh=1.0 # <--- ADDED
):
self.n = n
# Set neuron parameters
self.dim = dim # Dimensionality of the input
self.tau_rc = tau_rc # Membrane time constant
self.tau_ref = tau_ref # Refractory period
self.v_th = np.ones(n) * v_th # Threshold voltage for spiking
self.t_step = t_step # Time step for simulation
self.inh = np.zeros(n) # <--- ADDED
self.tau_inh = tau_inh # <--- ADDED
self.inc_inh = inc_inh # <--- ADDED
# Initialize state variables
# self.voltage = np.ones(n) * v_init # Initial voltage of neurons
self.voltage = np.random.uniform(0, 1, n) # Initial voltage of neurons
self.refractory_time = np.zeros(n) # Time remaining in refractory period
self.output = np.zeros(n) # Output spikes
# Generate random max rates and intercepts within the given range
max_rates_tensor = np.random.uniform(max_rates[0], max_rates[1], n)
intercepts_tensor = np.random.uniform(intercept_range[0], intercept_range[1], n)
# Calculate gain and bias for each neuron
# self.gain = self.v_th * (1 - 1 / (1 - np.exp((self.tau_ref - 1/max_rates_tensor) / self.tau_rc))) / (intercepts_tensor - 1)
# self.bias = np.expand_dims(self.v_th - self.gain * intercepts_tensor, axis=1)
self.gain = np.ones(n)
self.bias = np.zeros(n)
# Initialize random encoders
# self.encoders = np.random.randn(n, self.dim)
# self.encoders /= np.linalg.norm(self.encoders, axis=1)[:, np.newaxis]
self.encoders = np.ones((n, self.dim))
def reset(self):
# Reset the state variables to initial conditions
self.voltage = np.zeros(self.n)
self.refractory_time = np.zeros(self.n)
self.output = np.zeros(self.n)
self.inh = np.zeros(self.n) # <--- ADDED
def step(self, inputs):
dt = self.t_step # Time step
# Update refractory time
self.refractory_time -= dt
delta_t = np.clip(dt - self.refractory_time, 0, dt) # ensure between 0 and dt
# Calculate input current
I = np.sum(self.bias + inputs * self.encoders * self.gain[:, np.newaxis], axis=0) / self.n
# Update membrane potential
self.voltage = I + (self.voltage - I) * np.exp(-delta_t / self.tau_rc)
# Determine which neurons spike
spike_mask = self.voltage > self.v_th + self.inh # <--- ADDED + self.inh
self.output[:] = spike_mask / dt # Record spikes in output
# Calculate the time of the spike
t_spike = self.tau_rc * np.log((self.voltage[spike_mask] - I[spike_mask]) / (self.v_th[spike_mask] - I[spike_mask])) + dt
# Reset voltage of spiking neurons
self.voltage[spike_mask] = 0
# Set refractory time for spiking neurons
self.refractory_time[spike_mask] = self.tau_ref + t_spike
self.inh = self.inh * np.exp(-dt / self.tau_inh) + self.inc_inh * (self.output > 0) # <--- ADDED
return self.output # Return the output spikes
Show code cell source
import matplotlib.pyplot as plt
import json
import numpy as np
with open("../_static/datasets/xeae.json") as f:
xeae = np.array(json.loads(f.read()))
fig, axs = plt.subplots(NUM_NEURON_ROWS, NUM_NEURON_COLS, figsize=(8, 8))
for i in range(NUM_NEURONS):
ax = axs[i // NUM_NEURON_COLS, i % NUM_NEURON_COLS]
ax.imshow(xeae[:, i].reshape(DIGIT_WIDTH, DIGIT_HEIGHT), cmap="gray")
ax.axis("off")
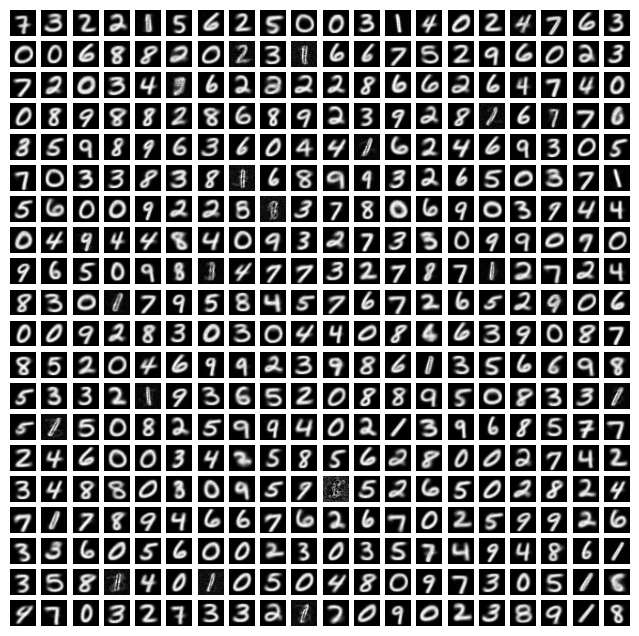
As you can see, most of these weights look like clearly discernable numbers. Each neuron is responsive to a particular digit (and a particularly way of drawing that digit). Each of these neurons is labeled according to the digit it responds to but we’ll pre-label them all.
We are also going to use pre-set firing thresholds for our excitatory neurons’ ALIFs. When we’re actually running our network, we will “freeze” both the STDP weights and the excitatory ALIFs’ firing thresholds.
Show code cell source
import zipfile
import json
import itertools
import random
from myst_nb import glue
random.seed(0)
def dataGenerator(path):
with zipfile.ZipFile(path) as train_zip:
with train_zip.open('index.json') as index_file:
idx_info = json.loads(index_file.read())
files = idx_info['files']
N = idx_info['N']
i = 0
for fname in files:
with train_zip.open(fname) as f:
data = json.loads(f.read())
images = data['images']
labels = data['labels']
for img, label in zip(images, labels):
yield (img, label)
i += 1
if i >= N: break
testDataGenerator = dataGenerator('../_static/datasets/test-chunked.zip')
Show code cell source
with open('../_static/datasets/theta.json') as theta_file:
theta = json.loads(theta_file.read())
with open('../_static/datasets/neuron_labels.json') as labels_file:
labels = json.loads(labels_file.read())
NUM_EXCITATORY = 400
TIME_TO_SHOW_IMAGES = 0.55 # seconds
TIME_TO_SHOW_BLANK = 0.15 # seconds
# print(xeae)
t_step = 0.001 # Time step for the simulation
synapses = SynapseCollection(n=DIGIT_SIZE, tau_s=0.1, t_step=t_step) # Synapse collection for input connections
# STDP (Spike-Timing-Dependent Plasticity) weight matrix between input and excitatory neurons
# stdp = STDPWeights(numPre=DIGIT_SIZE, numPost=NUM_EXCITATORY, g_min=-0.1, g_max=1.1)
# Inhibitory neurons and their corresponding post-synaptic potential (PSP) collection
inhibitory_neurons = LIF(n=NUM_EXCITATORY, t_step=t_step)
inhibitory_psp = SynapseCollection(n=NUM_EXCITATORY, t_step=t_step, tau_s=0.5)
inhibitory_outp = np.zeros(NUM_EXCITATORY) # Initialize inhibitory output array
# Excitatory neurons and their corresponding PSP collection
excitatory_neurons = ALIF(n=NUM_EXCITATORY, t_step=t_step, tau_inh=1.1)
excitatory_neurons.inh = np.array(theta)
excitatory_psp = SynapseCollection(n=NUM_EXCITATORY, t_step=t_step, tau_s=0.2)
# Function to perform a simulation step
def step(inp, max_input_rate):
global inhibitory_outp
input_spikes = poisson_fire(np.array(inp), dt=t_step, min_rate=0.01, max_rate=max_input_rate) # Generate input spikes
input_psp = synapses.step(input_spikes) # Step the input synapses to get PSP
# stdp.step(t_step) # Step the STDP mechanism
# stdp.updateWeights(input_spikes, excitatory_neurons.output) # Update weights based on input and output spikes
# uses xeae as weights rather than stdp (assuming fixed weights)
excitatory_inp = input_psp @ xeae + np.clip(inhibitory_outp * -1 / NUM_EXCITATORY, a_max=0, a_min=None) # Calculate excitatory input combining synapse output and inhibitory output
excitatory_spikes = excitatory_neurons.step(excitatory_inp) # Step excitatory neurons to get their spikes
excitatory_outp = excitatory_psp.step(excitatory_spikes) # Update the excitatory post-synaptic potential
inhibitory_spikes = inhibitory_neurons.step(excitatory_outp) # Step inhibitory neurons using excitatory output
# print(input_psp@stdp.w, excitatory_inp, excitatory_spikes, excitatory_outp, inhibitory_spikes)
raw_inhibitory_outp = inhibitory_psp.step(inhibitory_spikes) # Update the inhibitory post-synaptic potential
total_inhibitory_outp = np.sum(raw_inhibitory_outp) # Calculate the total inhibitory output
inhibitory_outp = total_inhibitory_outp * np.ones(NUM_EXCITATORY) - raw_inhibitory_outp # Calculate the inhibitory output for each neuron
return excitatory_spikes > 0
Then, we can run an example of our network classifying a new digit, highlighting the neuron(s) that fire most below (indicating that those are the neurons that “recognized” the digit).
Show code cell source
def run_simulation(num_images=1):
for _ in range(num_images):
current_image, label = next(testDataGenerator) # Get the next image and label from the data generator
total_fires = 0 # Initialize the total number of fires
max_input_rate = 0.2 # Initial maximum input firing rate
all_fires = np.zeros(NUM_EXCITATORY) # Initialize the array to store all the fires
max_fires = 0
has_dominant_neuron = False
while has_dominant_neuron == False and max_fires < 10:
for _ in np.arange(0, TIME_TO_SHOW_IMAGES, t_step): # Display the image for a set duration
fires = step(np.array(current_image), max_input_rate=max_input_rate) # Perform a simulation step
all_fires += fires # Accumulate the number of fires
num_fires = np.sum(fires > 0) # Calculate the number of fires
total_fires += num_fires # Accumulate the number of fires
max_input_rate *= 1.1 # Increase the input firing rate
max_fires = np.max(all_fires)
has_dominant_neuron = np.sum(all_fires == max_fires) <= 1 # Check if there is a dominant neuron
if has_dominant_neuron and max_fires >= 10: break
for _ in np.arange(0, TIME_TO_SHOW_BLANK, t_step): # Show a blank input for a set duration
step(np.zeros(len(current_image)), max_input_rate=max_input_rate) # Perform a simulation step with blank input
dominant_idx = np.argmax(all_fires)
predicted_label = labels[dominant_idx]
print(f"Predicted Label: {predicted_label}, True Label: {label}")
plt.figure()
plt.imshow(np.array(current_image).reshape(DIGIT_WIDTH, DIGIT_HEIGHT), cmap="gray")
plt.show()
fig, axs = plt.subplots(NUM_NEURON_ROWS, NUM_NEURON_COLS, figsize=(8, 8))
for i in range(NUM_NEURONS):
ax = axs[i // NUM_NEURON_COLS, i % NUM_NEURON_COLS]
ax.imshow(xeae[:, i].reshape(DIGIT_WIDTH, DIGIT_HEIGHT), cmap="gray" if all_fires[i] < max_fires else "hot")
ax.axis("off")
run_simulation()
def plotWeights():
NUM_DISPLAY_ROWS = 4
NUM_DISPLAY_COLS = 4
plt.figure()
for i in range(NUM_EXCITATORY):
plt.subplot(NUM_DISPLAY_ROWS, NUM_DISPLAY_COLS, i+1)
plt.imshow(stdp.w[:, i].reshape(DIGIT_WIDTH, DIGIT_HEIGHT), cmap='gray')
plt.axis('off')
plt.show()
Predicted Label: 7, True Label: 7
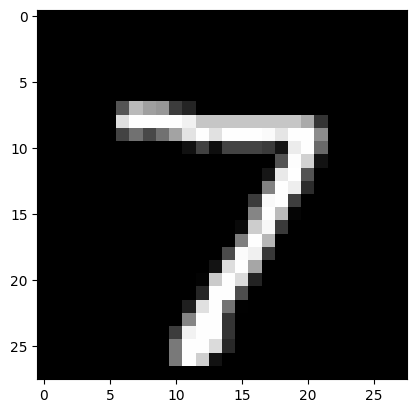
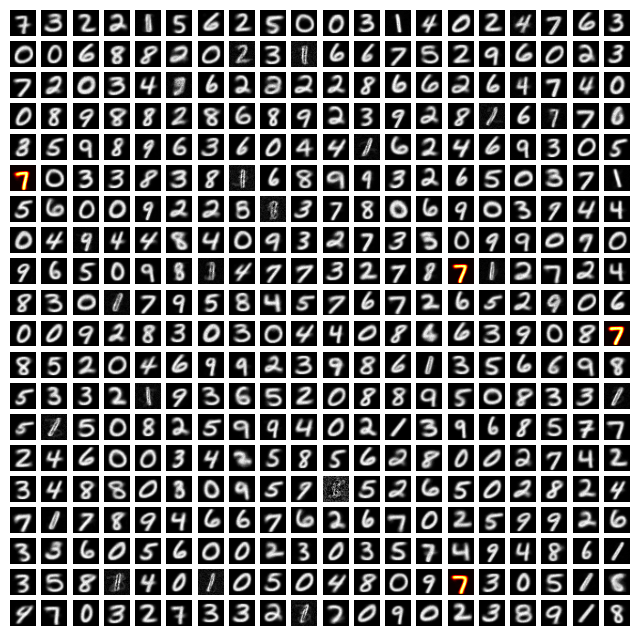
Our simulation worked well this time; the neurons that fired most were the ones that corresponded to the digit in the image (though the network is often inaccurate).
Summary#
We created a network that can recognize digits from the MNIST dataset
Our network uses primitives that we learned in prior notebooks: LIFs, ALIFs, STDP, and PSPs
The STDP weights, which we learn over time, encode the digits being recognized
Our network is a scaled down version of the one implemented by Diehl and Cook [DC15]
Note: This notebook (mostly) follows the paper: Diehl, Peter U., and Matthew Cook. “Unsupervised learning of digit recognition using spike-timing-dependent plasticity.” [DC15]